How To Find Gradient At Tangent
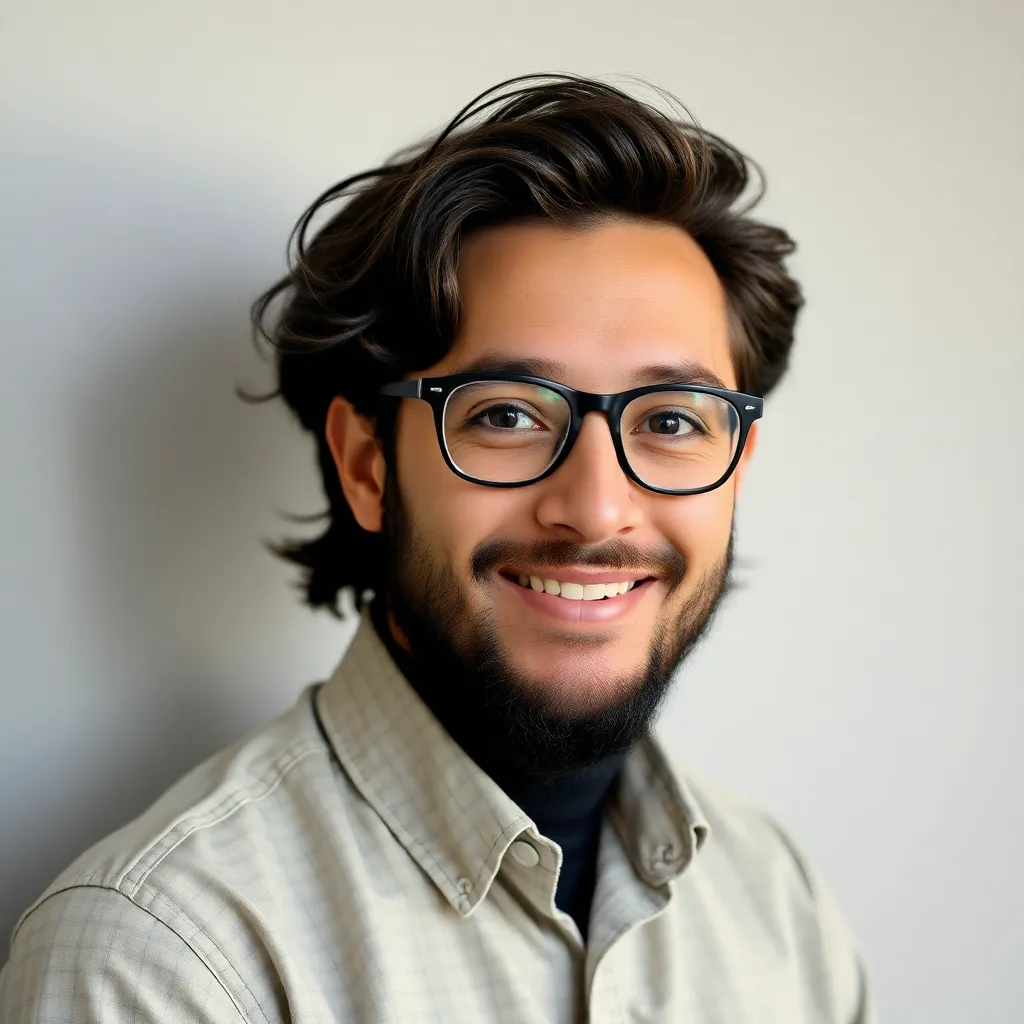
Ronan Farrow
Feb 24, 2025 · 3 min read
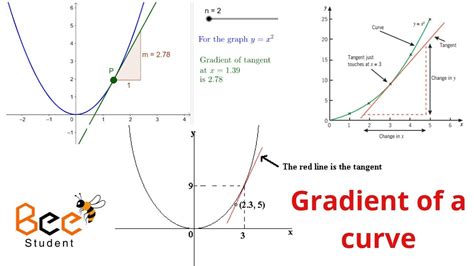
Table of Contents
How to Find the Gradient at a Tangent: A Comprehensive Guide
Finding the gradient at a tangent is a fundamental concept in calculus, crucial for understanding slopes of curves, rates of change, and optimization problems. This guide will walk you through the process, breaking it down into digestible steps, regardless of your current mathematical background.
Understanding the Gradient
The gradient at a point on a curve represents the instantaneous rate of change of the function at that specific point. Visually, it's the slope of the tangent line touching the curve at that point. A steeper tangent implies a larger gradient (a faster rate of change), while a flatter tangent suggests a smaller gradient (a slower rate of change).
Methods for Finding the Gradient
The most common method for finding the gradient at a tangent involves derivatives. The derivative of a function, f(x), denoted as f'(x) or df/dx, gives us a new function that represents the gradient at any point x.
1. Using Derivatives: The Power of Calculus
This is the most straightforward method. Let's illustrate with an example:
Example: Find the gradient of the function f(x) = x² + 2x + 1 at x = 2.
Steps:
-
Find the derivative: The derivative of f(x) = x² + 2x + 1 is f'(x) = 2x + 2 (using the power rule of differentiation).
-
Substitute the x-value: Substitute x = 2 into the derivative: f'(2) = 2(2) + 2 = 6.
Therefore, the gradient of the function at x = 2 is 6.
2. Using Numerical Methods (for complex functions)
When dealing with complex functions where finding an analytical derivative is difficult or impossible, numerical methods offer an approximation. These methods estimate the gradient by calculating the slope of a secant line between two closely spaced points on the curve.
Example (Approximation):
Let's use the same function f(x) = x² + 2x + 1, but approximate the gradient at x = 2 using a numerical method.
-
Choose a small increment (h): Let's use h = 0.001.
-
Calculate the function values:
- f(2) = 2² + 2(2) + 1 = 9
- f(2 + h) = f(2.001) = 2.001² + 2(2.001) + 1 ≈ 9.006001
-
Approximate the gradient: The gradient is approximately [f(2 + h) - f(2)] / h = (9.006001 - 9) / 0.001 ≈ 6.001.
This numerical approximation is very close to the exact value of 6 obtained using the derivative. Smaller values of h generally yield more accurate approximations.
Applications of Finding the Gradient at a Tangent
The ability to find the gradient at a tangent has wide-ranging applications in various fields:
- Optimization problems: Finding maxima and minima of functions.
- Physics: Calculating velocity and acceleration.
- Engineering: Designing optimal structures and systems.
- Economics: Modeling and predicting economic trends.
- Machine Learning: Gradient descent algorithms for training models.
Conclusion
Understanding how to find the gradient at a tangent is a key skill in calculus and its applications. Whether you use derivatives for exact solutions or numerical methods for approximations, mastering this concept opens doors to solving a wide array of challenging problems across numerous disciplines. Remember to practice regularly to solidify your understanding!
Featured Posts
Also read the following articles
Article Title | Date |
---|---|
How To Increase Testosterone Kids | Feb 24, 2025 |
How To Block Tiktok Account You Dont Follow | Feb 24, 2025 |
How To Yoga Journal | Feb 24, 2025 |
How To Lose Weight During Periods | Feb 24, 2025 |
How To Blur Whatsapp Web Safari | Feb 24, 2025 |
Latest Posts
-
How Long Is An Annulment
Apr 06, 2025
-
How Long Is An Acupuncture Needle
Apr 06, 2025
-
How Long Is Allegany Lpn To Rn Program
Apr 06, 2025
-
How Long Is A Wisdom Teeth Consultation
Apr 06, 2025
-
How Long Is A Trundle Bed
Apr 06, 2025
Thank you for visiting our website which covers about How To Find Gradient At Tangent . We hope the information provided has been useful to you. Feel free to contact us if you have any questions or need further assistance. See you next time and don't miss to bookmark.